How can AI and predictive analytics contribute to food safety? This is a question I keep asking myself and my colleagues for a long time now, trying to comprehend the versatility of food safety predictive analytics and find new ways in which we can use this technology to prevent risks from appearing in food supply chains, and make the world a safer place to eat.
It was about time to start broadening the conversation and ask this question to the people whose work can benefit most from food safety predictive analytics: the food safety leaders of the largest food manufacturing companies in the world.
The first that came to my mind was Zoltan Syposs, VP of Quality, Safety & Environment at The Coca-Cola Company, since we had already talked a lot about this topic earlier this year, when he joined a round-table discussion we organized at the GFSI21 in March and shared his opinion on the Promise and Perils of Predictive Analytics for Food Safety.
Holding a Ph.D. degree in Food Microbiology, Zoltan joined Coca-Cola in 2000 and is a proven global system leader with over 20 years of experience working across both The Coca-Cola Company and bottling operations. Apart from his corporate occupations, Zoltan’s also an Associate Professor at the Food Safety & Microbiology Department of the Szent Istvan University, Hungary, and recently he became a member of the GFSI Steering Committee.
His answers, along with many other interesting and thought-provoking ones from distinguished food safety leaders, are gathered in our latest discussion paper on Artificial Intelligence & Predictive Analytics for Food Risk Prevention.
Zoltan, what impact do you believe that Artificial Intelligence (AI) and predictive analytics are having on the food supply chain?
Tools, including those that are AI-based, clearly provide better consumer behavior predictions. By converting accurate consumer behavior predictions to leading SC key business indicators (KBI) we create a positive chain reaction across the food supply. More accurate raw material inventory, ingredient purchasing, and supply-and-demand monitoring help to cut down on excess inventories, reduce waste and improve productivity, thus improving cost structures.
Because AI is very good at dealing with a lot of variation and has machine vision and control, and can work non-stop, it is leading the way in next generation quality and food safety measures.
On the other hand, the increased complexity, demand for agility and resilience, mean that AI-driven, digitized food supply chains will continue to face both traditional and emerging risks, including cyber-security, food fraud and emerging contaminant related risks.
AI creates exciting opportunities for organizations to gain competitive advantage, but it also presents new opportunities for security attacks, thus posing significant challenges.
What are the critical food risk prevention questions that predictive analytics can help answer?
Predictive analytics will increasingly continue to play a role of paramount importance in quality and food safety. Critical food risk prevention questions that predictive analytics can help answer may include:
- Is this a risk for my business? If yes, why?
- Has this risk been addressed externally and/or within my SC? How is it being addressed?
- What impact will it have if not addressed?
Additionally…
- Where are my Supply Chain risks and how are they being managed?
- Where are my Innovation risks and how are they being managed?
- Where are my Customer risks and how are they being managed?
- What do I know and what do I need to know to make a decision?
What kind of public or private data assets will be required to train and feed such AI applications?
Prediction machines utilize three types of data: (1) AI training data; (2) prediction input data and (3) feedback data for improving prediction accuracy. I believe in the near future (3-5 years) we will see exponential improvements in both quality and pace related to Quality/Food Safety management.
- Supplier information (audit status, single sourced, lot-to-lot information, adherence to specification/lot testing results, supply disruptions, complaints/patterns on ingredients),
- Manufacturing (maintenance, process validation, training, internal/external audits, GMP assessments, cleaning & sanitation records, on-line monitoring, QC lab results, changeovers, internal & external failures, etc.),
- Distribution and marketplace (inventory, market velocity, product freshness, interactive labels),
- Innovation, including input to Quality by Design processes,
- Customer (requirements/expectations/emerging trends),
- Consumer (consumer response system/social media).
We need to enhance our competencies to respond to customers and keep up with consumer trends that increasingly require more transparency and enhanced communication.
By strengthening and further harmonizing food safety systems at scale and leveraging initiatives such as the GFSI “Race to the Top”, we can increase food safety capabilities across the global food supply chain and provide a platform for public-private partnerships.
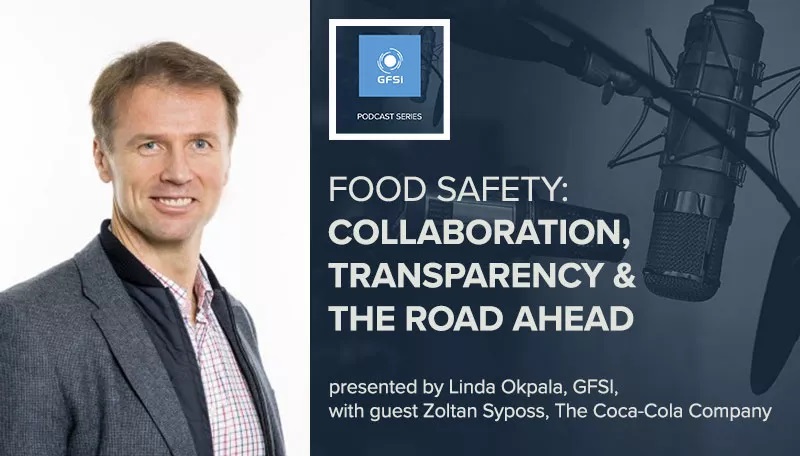
(GFSI podcast with Zoltan talking about the power of collaboration & transparency: https://mygfsi.com/podcast/food-safety-collaboration-transparency-and-the-road-ahead/)
Which factors will accelerate or impede the widespread adoption of AI and predictive analytics for food risk prevention and how does your company use this technology to make critical decisions?
Factors such as technology (data access) and computing power, the associated continually improving cost structures, and the quality and accessibility of trusted security solutions are just a few key components which will accelerate and impede the adoption of AI and predictive analytics.
We use both traditional (conventional) methods as well as predictive analytics. Science-based methods and advanced technologies help anticipate, assess, prevent, and manage food safety risks. Some of the traditional methods include consumer trends analysis, microbiological and toxicological reports, general applied food science, HACCP program advancement, and collaboration among industry and academia. It includes working closely with all key stakeholders and ensuring safety-by-design is embedded in our innovation programs.
The longer-term direction is toward a more multi-dimensional framework that can predict the impact discrete changes will have across our value chain. For example, how can an ingredient at a supplier increase or decrease the likelihood or impact on finished products across multiple regions? We are developing and integrating more predictive food safety analytics within our systems and programs. This is the result of deeper introspection into our internal processes and risks while simultaneously scanning the external world for current and future risks. By accelerating predictions, decision making, tool development and reduction in cost, we improve strategy and help society.
In my view, one of the fastest changes in this will be the transformation of applied QFS specifications. Traditional statistical methods require the articulation of hypotheses, or at least a human intuition for a risk assessment model specification. Machine learning does not require such specificity in advance and can accommodate the equivalent of much more complex models with many more interactions between variables.
Join this conversation. Sign up to the Interest Group on Predictive Analytics for Food Integrity, an industry group discussing innovative applications of predictive models for food risk prediction & prevention.